dr. Sofie Van Gassen (PhD)
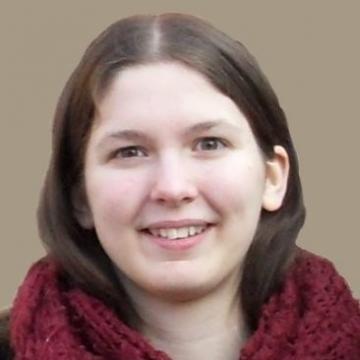
Postdoctoral researcher - Data Mining and Modelling for Biomedicine Group, Inflammation Research Center, UGent-VIB
Principal investigator: prof. Yvan Saeys (PhD)
Research focus
Flow cytometry is a unique technology which allows to measure characteristics of individual cells in a high-throughput setting (e.g. processing 10 000 cells / second, while measuring 10 to 50 properties per cell). This technology can give a detailed insight in the heterogeneity of tumor samples or allow follow-up of treatment. Especially in blood cancer research, flow cytometry is very valuable.
My research focuses on the development of computational techniques to aid in the analysis of flow cytometry data. Where traditional analysis becomes slow and biased towards expected cell populations for high-dimensional datasets, the use of algorithmic tools allows to apply a more comprehensive analysis. Our group has developed the FlowSOM algorithm, which can automatically identify cell populations, the FloReMi algorithm, which can be used to find correlations between identified populations and the patient survival time, the CytoNorm algorithm to correct batch effects and the PeacoQC algorithm to evaluate stability of the measurements over time. We are also developing pipelines to apply these algorithms on clinical datasets, collaborating with clinical researchers to gain deeper insights into the patient cohorts.
Biography
Sofie Van Gassen (°1990, Lokeren, Belgium) received her M.S. degree in Computer Science from Ghent University in 2013 and her PhD in Computer Science Engineering from Ghent University in 2017. During her PhD she developed machine learning techniques for flow and mass cytometry data. This included the FlowSOM algorithm, a well-known clustering tool for flow cytometry data. She also participated in the FlowCAP IV challenge, where the FloReMi pipeline got the best results in predicting progression time to AIDS for HIV patients. From 2018 to 2023, she was an ISAC Marylou Ingram Scholar. Currently, she is further extending and improving machine learning techniques for single cell data as a postdoc in the DaMBi group (VIB-UGent Center for Inflammation Research).
Key publications
'Analyzing high-dimensional cytometry data using FlowSOM'. Nature Protocols,. 2021.
'CytoNorm: A Normalization Algorithm for Cytometry Data'. Cytometry, 2019.
‘Myeloid cell heterogeneity in cancer: not a single cell alike’. Cellular immunology, 2018. (PMID: 29482836)
'FlowSOM: Using self-organizing maps for visualization and interpretation of cytometry data'. Cytometry, 2015.
Contact & links
- Lab Address: Data Mining and Modelling for Biomedicine Group, Inflammation Research Center, UGent-VIB, Technologiepark 71, B-9052 Gent, Belgium
- Data Mining and Modelling for Biomedicine Group